Sliding Puzzles Gym: A Scalable Benchmark for State Representation in Visual Reinforcement Learning
SPGym extends the 15-tile puzzle to evaluate RL algorithms by scaling representation learning while keeping latent dynamics and algorithmic problem fixed, revealing opportunities for advancing representation learning for decision-making research.

Abstract
Learning effective visual representations is crucial in open-world environments where agents encounter diverse and unstructured observations. This ability enables agents to extract meaningful information from raw sensory inputs, like pixels, which is essential for generalization across different tasks. However, evaluating representation learning separately from policy learning remains a challenge in most reinforcement learning (RL) benchmarks. To address this, we introduce the Sliding Puzzles Gym (SPGym), a benchmark that extends the classic 15-tile puzzle with variable grid sizes and observation spaces, including large real-world image datasets. SPGym allows scaling the representation learning challenge while keeping the latent environment dynamics and algorithmic problem fixed, providing a targeted assessment of agents' ability to form compositional and generalizable state representations. Experiments with both model-free and model-based RL algorithms, with and without explicit representation learning components, show that as the representation challenge scales, SPGym effectively distinguishes agents based on their capabilities. Moreover, SPGym reaches difficulty levels where no tested algorithm consistently excels, highlighting key challenges and opportunities for advancing representation learning for decision-making research.
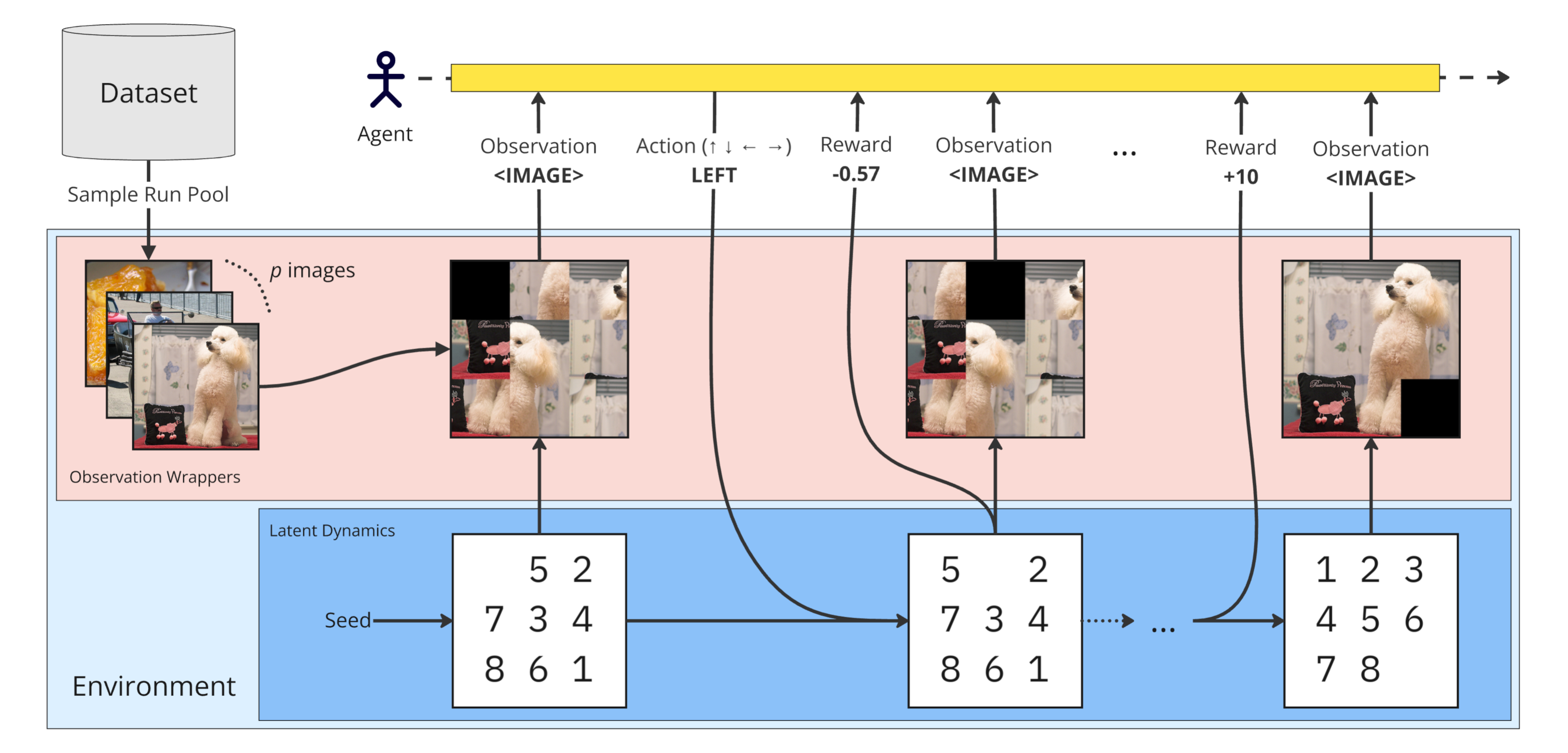
Overview of the Sliding Puzzles Gym (SPGym). The framework extends the 15-tile puzzle by incorporating image-based tiles, allowing scalable representation complexity while maintaining fixed environment dynamics.
Poster at the Workshop on Open-World Agents at the 38th Conference on Neural Information Processing (NeurIPS 2024).